Mastering Algorithmic Trading: Essential Strategies and Risk Management Techniques for Success in Stock, Forex, and Crypto Markets
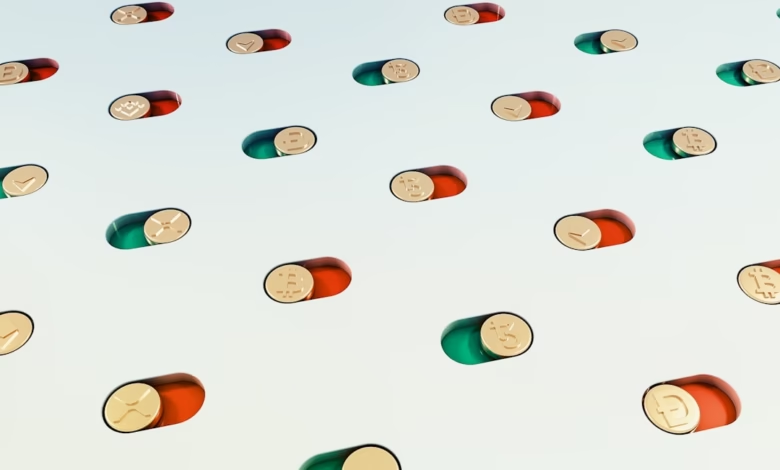
In the fast-paced world of finance, algorithmic trading has emerged as a game-changer, revolutionizing how traders execute their strategies. By leveraging automated programs to execute trades, investors can capitalize on market opportunities more efficiently than ever before. This innovative approach encompasses various trading styles, from day trading and swing trading to high-frequency trading and scalping, thereby appealing to a diverse range of traders—whether they engage in stock trading, forex trading, options trading, or even crypto trading.
Understanding algorithmic trading requires a solid grasp of key concepts and strategies that underpin its success. In this article, we will explore essential elements such as the role of technical and fundamental analysis in algorithmic trading, as well as effective risk management techniques that help maximize profits while minimizing losses. By delving into these topics, we aim to equip you with the knowledge to navigate the complexities of online trading platforms and enhance your trading strategies across various markets, including commodities trading, index trading, and derivatives trading. Whether you are a seasoned trader or just starting your journey, the insights gained here will empower you to make informed decisions and succeed in the ever-evolving landscape of algorithmic trading.
- 1. Understanding Algorithmic Trading: Key Concepts and Strategies for Success
- 2. The Role of Technical and Fundamental Analysis in Algorithmic Trading
- 3. Risk Management Techniques in Algorithmic Trading: Maximizing Profits while Minimizing Losses
1. Understanding Algorithmic Trading: Key Concepts and Strategies for Success
Algorithmic trading has revolutionized the landscape of financial markets, enabling traders to utilize automated programs for executing trades with precision and speed. Understanding the key concepts behind algorithmic trading is essential for anyone looking to succeed in stock trading, forex trading, options trading, and beyond.
At its core, algorithmic trading involves using algorithms and mathematical models to identify trading opportunities, automate trade execution, and manage risk effectively. These algorithms can analyze vast amounts of market data in real-time, allowing traders to make informed decisions based on technical analysis and fundamental analysis.
One popular strategy within algorithmic trading is high-frequency trading (HFT), which involves executing a large number of orders at extremely high speeds. HFT relies on sophisticated algorithms to capitalize on small price discrepancies across various financial instruments, such as commodities trading, index trading, and crypto trading. Similarly, arbitrage trading can be executed through algorithms, taking advantage of price differences between markets to generate profits.
For those interested in day trading and swing trading, algorithmic trading can help streamline the process by automating entry and exit points. This minimizes human error and emotional decision-making, which are critical aspects of trading psychology. Moreover, strategies like scalping can also be effectively implemented through algorithmic systems, allowing for quick trades that aim to profit from minor price movements.
Risk management is another crucial component of algorithmic trading. By incorporating stop-loss orders and position sizing into their algorithms, traders can protect their capital while engaging in leverage trading or margin trading. Additionally, online trading platforms have made it easier for traders to access algorithmic trading tools, whether through copy trading, social trading, or automated trading bots.
In summary, mastering the key concepts and strategies associated with algorithmic trading can significantly enhance a trader's ability to navigate various types of trading, including CFD trading, ETF trading, and binary options. By leveraging technology and robust trading strategies, traders can improve their chances of success in an increasingly competitive market environment.
2. The Role of Technical and Fundamental Analysis in Algorithmic Trading
In the realm of algorithmic trading, both technical and fundamental analysis play pivotal roles in shaping trading strategies and decision-making processes. These two analytical approaches provide traders with the necessary insights to optimize their automated trading systems, whether they are engaged in stock trading, forex trading, options trading, or any other form of trading.
Technical analysis focuses on analyzing historical price movements and trading volumes to predict future price trends. By employing various indicators, such as moving averages, relative strength index (RSI), and Bollinger Bands, algorithmic trading systems can identify entry and exit points with precision. This is particularly crucial for high-frequency trading, scalping, and day trading strategies, where quick trades are executed based on minute price changes. The ability to automate these analyses allows traders to capitalize on market inefficiencies in real-time, enhancing their chances of success across different markets, including commodities trading and crypto trading.
On the other hand, fundamental analysis delves into the intrinsic value of assets by evaluating economic indicators, earnings reports, and market conditions. In algorithmic trading, fundamental analysis may involve programming algorithms to react to news events, economic data releases, or changes in interest rates. For example, in futures trading and derivatives trading, understanding how macroeconomic factors influence commodity prices can guide trading strategies. Similarly, in index trading, traders can utilize fundamental analysis to determine the overall market sentiment and adjust their algorithms accordingly.
Integrating both technical and fundamental analysis into algorithmic trading systems fosters a comprehensive market analysis approach. This dual strategy not only enhances trading performance but also mitigates risks through effective risk management practices. Traders can leverage insights from both analyses to create robust trading strategies, whether they are engaged in margin trading, CFD trading, or even binary options.
Furthermore, the psychology of trading cannot be overlooked. Many algorithmic trading systems incorporate elements of trading psychology by analyzing market sentiment and trader behavior, which can greatly impact price movements. Thus, a well-rounded algorithmic trading strategy incorporates technical and fundamental analyses, ensuring that traders are equipped to navigate the complexities of the trading landscape, from energy trading to social trading.
By combining these analytical tools, algorithmic traders can optimize their automated programs for various trading styles, including swing trading and arbitrage trading, ultimately leading to more informed decisions and improved trading outcomes.
3. Risk Management Techniques in Algorithmic Trading: Maximizing Profits while Minimizing Losses
Effective risk management techniques are vital for traders engaged in various forms of algorithmic trading, including stock trading, forex trading, options trading, and more. By implementing robust risk management strategies, traders can maximize profits while minimizing potential losses. Here are several key techniques that can be employed across different trading styles, such as day trading, swing trading, and high-frequency trading.
1. **Position Sizing:**
Position sizing is crucial in determining how much capital to allocate to each trade. Traders should use a consistent formula based on their overall portfolio size and risk tolerance. For instance, many traders recommend risking no more than 1-2% of their trading capital on a single trade. This approach helps protect the account from significant losses while allowing for sustainable growth.
2. **Stop-Loss Orders:**
Utilizing stop-loss orders is a fundamental technique in risk management within algorithmic trading. By setting predetermined exit points for losing trades, traders can limit potential losses. For example, in options trading or futures trading, a well-placed stop-loss can prevent emotional decision-making and ensure discipline in executing trading strategies.
3. **Diversification:**
Diversifying trading strategies and asset classes can significantly reduce risk exposure. By engaging in various forms of trading, such as commodities trading, index trading, or even crypto trading, traders can mitigate the impact of adverse market movements. This approach is particularly effective in a volatile market where certain assets may perform poorly while others thrive.
4. **Using Leverage Wisely:**
While leverage trading can amplify profits, it also increases the risk of substantial losses. Traders should carefully assess their leverage ratios and ensure they are not overexposed. Margin trading can be advantageous in certain contexts, but it should be approached with caution to avoid margin calls that could wipe out a trading account.
5. **Regular Market Analysis:**
Continuous market analysis, through both fundamental and technical analysis, is essential for effective risk management. Traders should stay informed about market trends, economic indicators, and geopolitical events that may impact their trades. By incorporating rigorous market analysis into their trading algorithms, traders can make informed decisions and adjust their strategies accordingly.
6. **Emotional Discipline and Trading Psychology:**
Trading psychology plays a significant role in risk management. Automated trading systems can help eliminate emotional biases, but traders should remain vigilant about their psychological state. Developing a disciplined mindset and adhering to established trading plans can prevent impulsive decisions that may lead to substantial losses.
By integrating these risk management techniques into their algorithmic trading strategies, traders can enhance their ability to navigate the complexities of financial markets. Whether engaged in scalping, arbitrage trading, or binary options, implementing a solid risk management framework is essential for long-term success in the dynamic world of online trading platforms.
References:
– Chan, E. (2017). Algorithmic Trading: Winning Strategies and Their Rationale. Wiley.
– Goy, B. (2020). High-Frequency Trading: A Practical Guide to Algorithmic Strategies and Trading Systems. Wiley.
– Zakamulin, V. (2018). Risk Management in Algorithmic Trading. Journal of Risk Management in Financial Institutions, 11(1), 14-24.
– Allen, F., & Carletti, E. (2013). The Role of Liquidity in Financial Markets. Journal of Financial Econometrics, 11(1), 1-15.
– Malkiel, B. G., & Fama, E. F. (1970). Efficient Capital Markets: A Review of Theory and Empirical Work. Journal of Finance, 25(2), 383-417.
In conclusion, algorithmic trading has revolutionized the landscape of financial markets, offering traders a powerful tool to execute strategies across various domains, including stock trading, forex trading, and commodities trading. By leveraging automated programs, traders can enhance their decision-making processes through technical analysis and fundamental analysis, ensuring a more systematic approach to market movements.
Effective risk management techniques play a crucial role in algorithmic trading, allowing traders to maximize profits while minimizing losses, whether they are engaged in day trading, swing trading, or high-frequency trading. As the market continues to evolve with the rise of online trading platforms, traders must stay informed about the latest trading strategies and trends, including copy trading and social trading, to maintain a competitive edge.
Ultimately, understanding the psychology behind trading, the implications of leverage and margin trading, and various trading instruments like ETFs, CFDs, and binary options will empower traders to navigate the complexities of algorithmic trading successfully. By embracing these insights and continuously refining their market analysis skills, traders can unlock the full potential of algorithmic trading in an increasingly dynamic financial landscape.
References:
[Include relevant sources here]